SaaSy AI? - Week in Review 19/02
The economics of AI-driven businesses and James Taylor's reflections
What’s up everyone? Not much to report this week. Do check out my pal Dennis Müller’s productivity library here. It was Product of the Day yesterday (18/02) on Product Hunt. Nice one guys!
Are AI and SaaS business models compatible?
On The New Business of AI (and How It’s Different From Traditional Software) by Martin Casado & Matt Bornstein
In the eyes of the a16z crew, AI companies differ from SaaS companies in three distinct ways.
For one, due to infrastructure costs and the necessity of human support, AI companies typically have gross margins in the realm of 50-60%, compared to the 70-80% meat-and-potatoes business software enjoys. Simply put, AI server costs are quite steep. Most AI models are trained on ‘rich media’ (i.e. video, sound, images etc.), which consumes significant storage resources and leads to more complex calculations, requiring more robust computing power. Casado estimates that up to 25% of revenues of AI concerns goes to infrastructure costs. Compounding the problem, requirements for processing power are increasing much faster than cutting-edge infrastructure can support.
Casado writes, “the compute resources required to train state-of-the-art AI models has grown over 300,000x since 2012, while the transistor count of NVIDIA GPUs has grown only ~4x!”
On top of insufficient infrastructure contributing to weak gross margins, the necessity of human oversight and participation in the training of AI models holds AI businesses back from capturing SaaS-like economics. On the one hand, humans must clean and label large data sets to properly train the AI models. Further, this work is continuous throughout the training period of the models; it is by no means ‘one-and-done’. Casado estimates that AI companies spend between 10-15% of revenue on organizing data sets alone. On top of organizing data, for many AI projects, humans need to be plugged-in in real time to act as joint decision makers. Unfortunately, the limitations of AI technology are now being fully understood and, thus, humans must interfere more than previously thought. On top of that, it is rather likely that human oversight of AI technology will be enshrined into government regulations, perhaps permanently dooming AI projects to sub-SaaS gross margins.
The second of the three differences lies in the prevalence of edge cases in customer usage of AI products. Unlike SaaS sales, in which on-boarding and training usually follows a rather repeatable playbook, deploying AI projects often results in unique outcomes. These unpredictable situations require considerable manpower to manage. Indeed, especially for an early-stage company, the necessity of overseeing deployments ties up both human and financial resources that could be better allocated to R&D or making new sales.
Casado summarizes how SaaS and AI businesses differ with edge cases well: “Like traditional software, the process is especially time-consuming with the earliest customer cohorts, but unlike traditional software, it doesn’t necessarily disappear over time.” Clearly, scaling is quite a bit more challenging for AI plays than for business software projects.
There is, more or less, a consensus on how to build strong moats in software. Network effects, high switching costs, and economies of scale are the building blocks of defensibility. More so, the best thing to have is a superior technological innovation. This, however, is difficult for AI companies to achieve. Casado explains:
“In the AI world, technical differentiation is harder to achieve. New model architectures are being developed mostly in open, academic settings. Reference implementations (pre-trained models) are available from open-source libraries, and model parameters can be optimized automatically. Data is the core of an AI system, but it’s often owned by customers, in the public domain, or over time becomes a commodity.”
There is even strong evidence suggesting that AI companies suffer from diseconomies of scale. Another a16z essay points out that as AI models grow, it becomes costlier to address edge cases while simultaneously providing weaker value for users over time. Casado concludes the section by pondering whether or not the actual AI models of companies will be defensible, remarking that instead the underlying product or data could provide a deep-enough moat. Prescriptively, Casado suggests that AI companies should aggressively vet their new customers and be willing to operate with hybrid software-services business models. This is not completely satisfying, however, as the implication of this strategy would lead to disappointing gross margins, at least comparable to software concerns.
Matt Turck, of FirstMark Capital and a well-regarded investor in AI projects, weighed in with his own take. He notes that AI companies can, over time, scale successfully to look more like SaaS, with gross margins around 80% and so on. He points out that there have been quite a few companies who have succeeded in externalizing the costs of servicing the AI models to customers, noting that clients feel empowered when allowed to tinker with the AI directly. He concludes that he is in fact seeing data network effects, though his argument that it leads to defensibility from other AI projects is hard to believe in for the long term.
To me, these particular problems seem to have a rather straightforward (at least from a theoretical perspective) solution: just like Amazon and Microsoft built massive businesses providing the infrastructure layer for SaaS to run on, there looks to be a significant opportunity for players, big and small, to build the rails for AI companies to construct their models on top of. In the words of another AI investor, “this [AI] stack is going to mature and is iterating rapidly”. Further, AI-driven companies can find other ways to build moats by leveraging different product lines. The same investor notes that technologically advanced hardware, upon which AI models run, can provide as much as a two year advantage to startups in the AI space. Taking it one more step, a dominant hardware player can expect to build not just a moat but a castle if they can also nail developing operational toolsets/pipelines alongside superior hardware. A big ask, for sure, but not an impossible one.
Thank you to Moritz Mueller-Freitag of TwentyBN for his assistance with this post.
You’ve Got a Friend
Check out Jenny Stevens’ excellent interview with James Taylor in the Guardian. He talks about being the first artist signed to The Beatles’ record label Apple Records, his difficulties kicking heroin and how his childhood shaped him into the adult he ended up being.
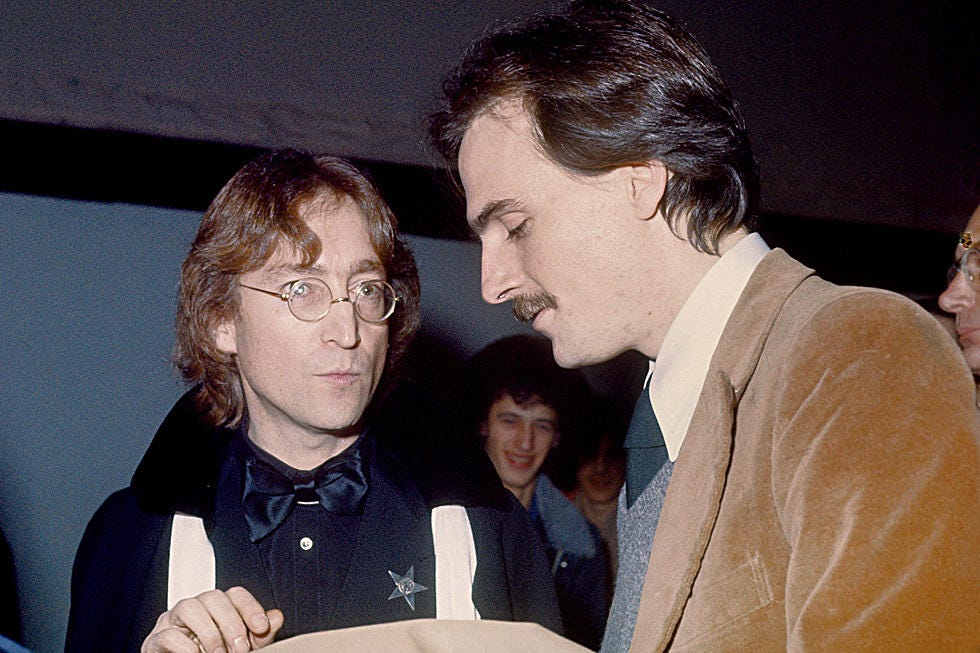
The themes of his music are eternal: “the precarity of our emotional lives, happiness as something to be treasured and the natural world’s capacity for renewal”. Listening to him, I’m always transported to Massachusetts in the winter time. Love him.
Here’s a good track for the uninitiated.
Soft rock forever!
Max